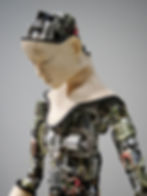
Nearly every product these days needs to feature Artificial intelligence (AI). We've been training data to detect anomolies in the authentication process, have better randomized password suggestions, and automation the creation of, well, automations. We've also been using modern AI techniques to make us faster at what we do, which lets us run with smaller teams and get more done. We can't just feed an AI our API and have it spit out swagger docs or write endpoints just yet (at least not if we want them to be secure and efficient), but we can augment our efforts and do.
One thing has become clear: AI is becoming increasingly sophisticated, and with that comes new challenges. One of the most concerning challenges is the potential for AI to hallucinate. An AI hallucination is a confident response by an AI that does not seem to be justified by its training data. This can happen when an AI is given too much data or when it is trained on data that is biased or inaccurate.
AI hallucinations can be dangerous. For example, an AI that is used to make medical diagnoses could provide incorrect information, which could lead to harm to patients. An AI that is used to make financial decisions could make bad investments, which could cost people money. Overly simplistic models could be used to game systems, but overly complex models can effectively break an algorithm, or deep learning paradigm.
There are a number of things that can be done to prevent AI hallucinations. One is to carefully curate the data that is used to train AI models. This means ensuring that the data is accurate and unbiased, even if that data is still unstructured. AI hallucinations are often caused by bias in the training data. This can happen when the training data is not representative of the real world, or when it contains harmful stereotypes.
AI hallucinations can also be caused by overfitting. This happens when an AI model learns the training data too well, and it starts to generate responses that are not actually true. Another way to prevent AI hallucinations is to use a technique called "data augmentation." This involves artificially expanding the training data by adding variations to it. This can help to prevent AI models from overfitting to the training data, which can lead to hallucinations.
Finally, it is important to monitor AI models for signs of hallucination. This can be done by looking for patterns in their responses that are not consistent with their training data.
By taking these steps, we can help to prevent AI hallucinations and ensure that AI is used safely and responsibly.
In short, we don't want to give Secret Chest users a false sense of security that we're detecting anomolies with a given degree of certainty. Nor do we want to surface a typical activity that appears as anomolous due to overfitting. Therefore, just know we're doing stuff in the background, and doing our best. However, you might not see it in marketing assets until we're pretty darn sure that we're hitting the mark!
In the meantime, if you'd like to use the product and so help us to train our models, please feel free to sign up to the Secret Chest private beta. We'd be lucky to have ya'.